Computer
Vision Project 1: Feature Detection and Matching
Md Tanvir Islam Aumi (tanvir@cs.washington.edu)
Feature
Descriptor
I
have implemented two types of feature descriptors. First
one is a simple one which just takes a 5x5 window around the harris
point. Second one is a more sophisticated one and more invariant to
rotation, illumination, and
scaling. The basic
steps for creating this feature
descriptor is described below:
Major
Design Choices
Throughout
the whole assignment, I had to deal with the
Accuracy-Runtime
trade-off. For
example, while calculating the local maxima of harris points, I only
chose those points that are above a particular
threshold.
If this threshold is
too low, then I have to deal with a lot of harris points which
increases runtime. However, the more the number of harris points, the
more the matching accuracy. So, I chose a threshold which maintains a
good
balance
between
accuracy
and runtime.
Another major design issue was the feature descriptor algorithm. I
tried scaling the 40x40 window into multiple sizes and stack them one
after another as a pyramid. However, it increases the runtime hugely.
Therefore, I scaled
it to one size (8x8) and it worked well with a reasonable runtime.
Performance
Performances (in terms of ROC curve) of different feature
descriptors and matching algorithms on two benchmark datasets are given
below (Figure 1a and 1b). X-axis is the FP rate and Y-axis is the
TP-rate. As expected, "MOPS + Ratio test" does the best job on both
datasets. Using this, we can select a reasonably well threshold which
gives a good TP rate with relatively low FP rate.
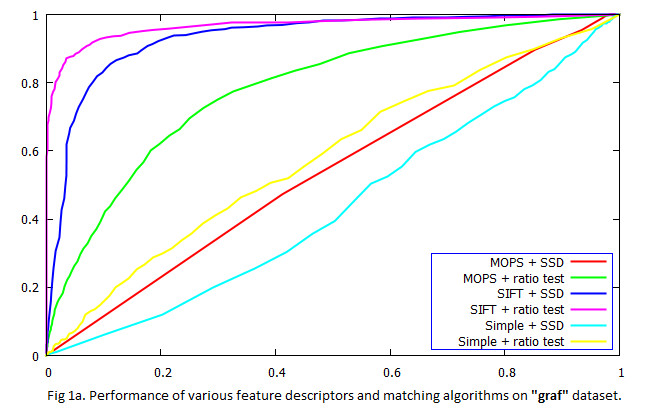
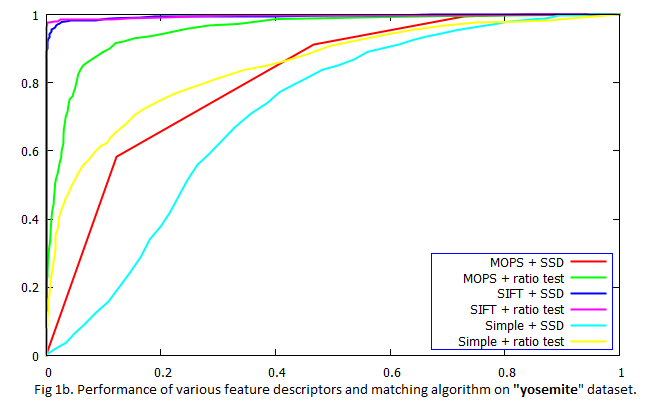
Another way of choosing threshold is to plot the (TP rate - FP rate) Vs
Threshold (Figure 2a and 2b). Using this plots, we can select the
X-value of the peak as a good measure of the threshold.
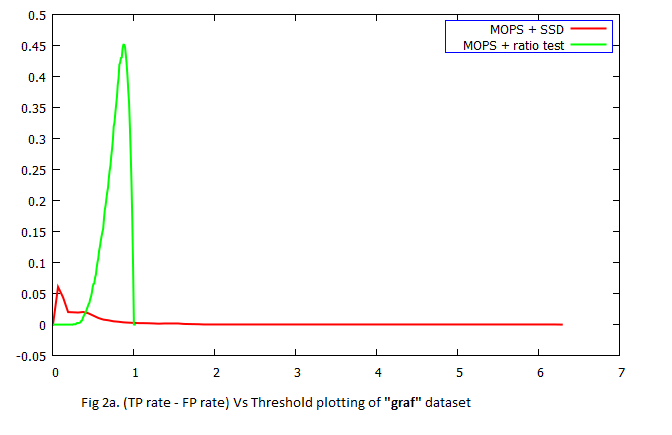
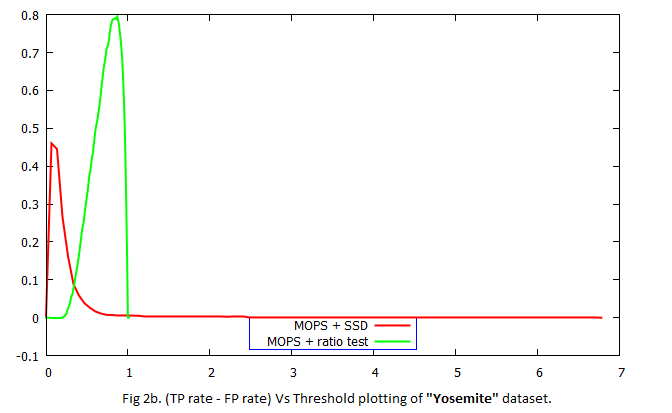
Performance of two descriptors (
Simple and MOPS) with two different
matching approaches (
SSD and Ratio)
on all the benchmark datasets are given below in Table 1.
|
Bikes |
Graf |
Leuven |
Wall |
Average
Pixel Error |
Average
AUC |
Average
Pixel Error |
Average
AUC |
Average
Pixel Error |
Average
Error |
Average
Pixel Error |
Average
AUC |
MOPS
+ Ratio |
389.26 |
0.77 |
268.92 |
0.70 |
160.45 |
0.85 |
295.42 |
0.85 |
MOPS
+ SSD |
389.26 |
0.58 |
268.92 |
0.48 |
160.45 |
0.60 |
295.42 |
0.54 |
Simple
+ Ratio |
397.63 |
0.46 |
313.00 |
0.56 |
383.09 |
0.53 |
382.46 |
0.61 |
Simple
+ SSD |
397.63 |
0.23 |
313.00 |
0.45 |
383.09 |
0.08 |
382.46 |
0.23 |
Table 1: Performace on all the benchmark datasets.
Harris Images
These are the sample harris images of two benchmark images.
To get a good brightness, original pixel values are multiplied by 1000
here.
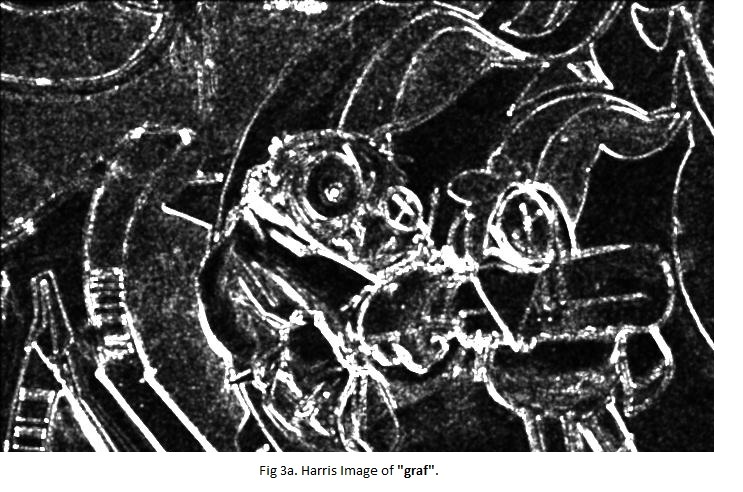