Testing Recognition with cropped class images (eig 25 x 25)
Average Face : |
|
Eigen Face 1 : |
|
Eigen Face 2 : |
|
Eigen Face 3 : |
|
Eigen Face 4 : |
|
Eigen Face 5 : |
|
Eigen Face 6 : |
|
Eigen Face 7 : |
|
Eigen Face 8 : |
|
Eigen Face 9 : |
|
Eigen Face 10 : |
|
Accuracies
Number of eigenfaces | Percent Correct | Fraction |
1 | | 18.5185 | 5/27 |
2 | | 44.4444 | 12/27 |
3 | | 48.1481 | 13/27 |
4 | | 48.1481 | 13/27 |
5 | | 55.5556 | 15/27 |
6 | | 48.1481 | 13/27 |
7 | | 51.8519 | 14/27 |
8 | | 66.6667 | 18/27 |
9 | | 62.9630 | 17/27 |
10 | | 74.0741 | 20/27 |
11 | | 77.7778 | 21/27 |
12 | | 74.0741 | 20/27 |
13 | | 74.0741 | 20/27 |
14 | | 74.0741 | 20/27 |
15 | | 74.0741 | 20/27 |
16 | | 74.0741 | 20/27 |
17 | | 77.7778 | 21/27 |
18 | | 74.0741 | 20/27 |
19 | | 74.0741 | 20/27 |
20 | | 74.0741 | 20/27 |
21 | | 74.0741 | 20/27 |
22 | | 74.0741 | 20/27 |
23 | | 74.0741 | 20/27 |
24 | | 74.0741 | 20/27 |
25 | | 74.0741 | 20/27 |
26 | | 74.0741 | 20/27 |
27 | | 74.0741 | 20/27 |
Generally, the results improve with the number of eigenvectors used, although it is noted that after about 10 eigenvectors, the results stop improving. A balance must be struck between the benefit of adding another eigenvector, which adds to the runtime of the algorithm, and the accuracy of the system. In this case, a good tradeoff value (that does not seem to be relying on the eccentricity of this particular data set, and hence is not an overfit) is 10 eigenvectors.
Here are some images of some mistakes (10 eigvecs)
Smiling Image | Classified as |
|  |
| 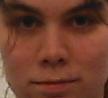 |
| 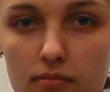 |
| 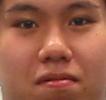 |
| 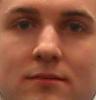 |
| 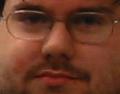 |
|  |
| 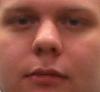 |
| 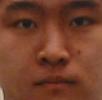 |
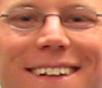 | 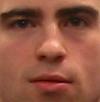 |
 |  |
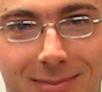 | 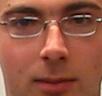 |
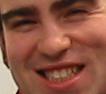 | 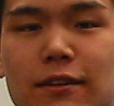 |
 |  |
 | 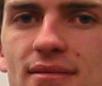 |
 |  |
 |  |
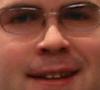 | 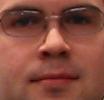 |
 |  |
 |  |
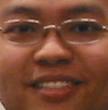 | 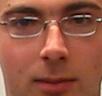 |
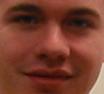 |  |
 |  |
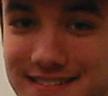 | 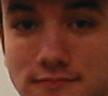 |
 |  |
 | 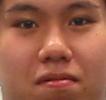 |
 |  |
The mistakes are somewhat reasonable as they look a little similar.
Cropping and finding faces
ELF Results | 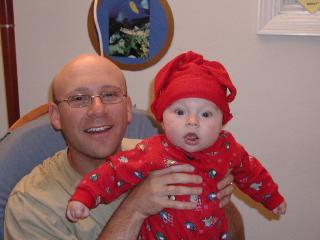 |  |
Portrait (min=0.3;max=0.7;step=0.05) | 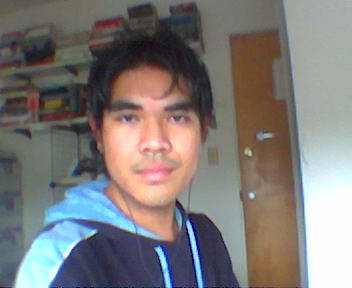 |  |
IMG_0031 (min=0.5;max=0.7;step=0.05) | 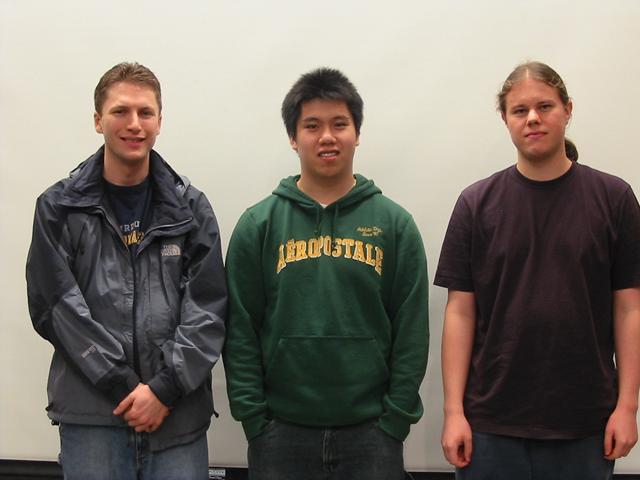 | 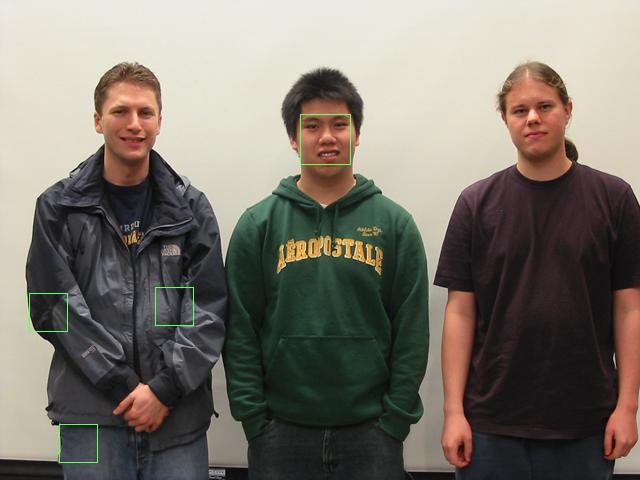 |
group2 (min=0.05;max=0.35;step=0.05) | 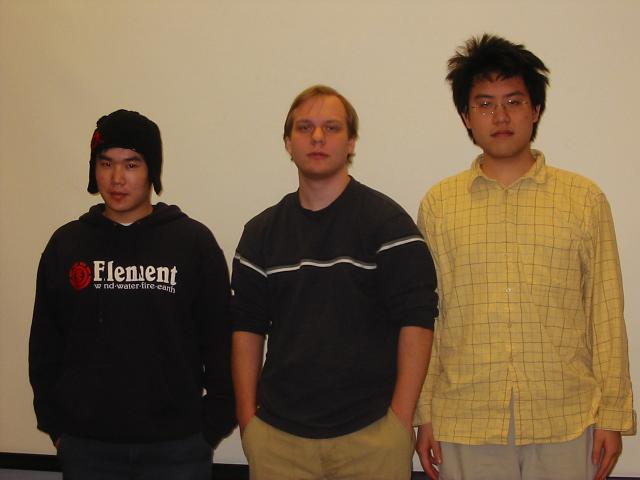 | 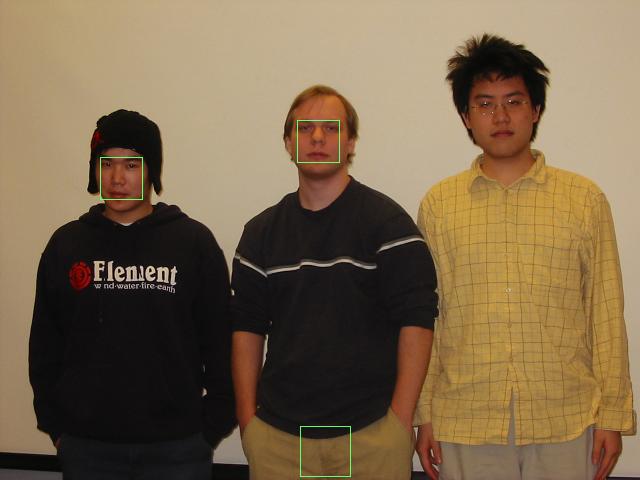
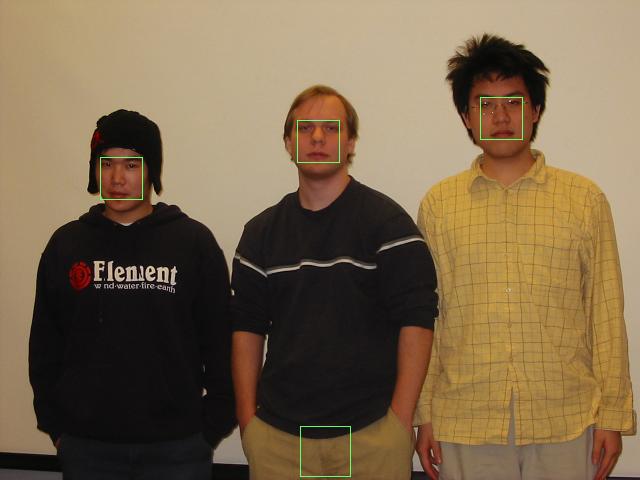 |
There were a lot of false positives in this case, possibly due to bugs and limitations of the features used.